Vector stores
As generative AI applications continue to push the boundaries of what’s possible in tech, vector stores have emerged as a crucial component, streamlining and optimizing the search and retrieval of relevant data. In our previous discussions, we’ve delved into the advantages of vector DBs over traditional databases, unraveling the concepts of vectors, embeddings, vector search strategies, approximate nearest neighbors (ANNs), and similarity measures. In this section, we aim to provide an integrative understanding of these concepts within the realm of vector DBs and libraries.
The image illustrates a workflow for transforming different types of data—Audio, Text, and Videos— into vector embeddings.
- Audio: An audio input is processed through an “Audio Embedding model,” resulting in “Audio vector embeddings.”
- Text: Textual data undergoes processing in a “Text Embedding model,” leading to “Text vector embeddings.”
- Videos: Video content is processed using a “Video Embedding model,” generating “Video vector embeddings.”
Once these embeddings are created, they are subsequently utilized (potentially in an enterprise vector database system) to perform “Similarity Search” operations. This implies that the vector embeddings can be compared to find similarities, making them valuable for tasks such as content recommendations, data retrieval, and more.
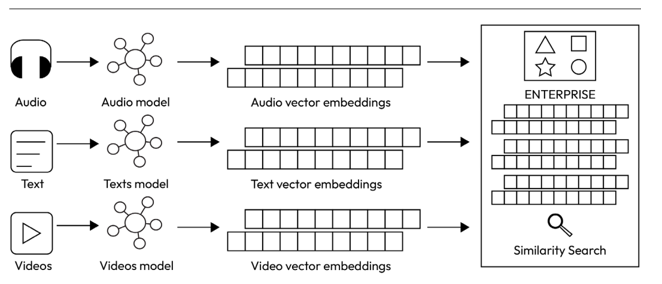
Figure 4.9 – Multimodal embeddings process in an AI application
What is a vector database?
A vector database (vector DB) is a specialized database designed to handle highly dimensional vectors primarily generated from embeddings of complex data types such as text, images, or audio. It provides capabilities to store and index unstructured data and enhance searches, as well as retrieval capabilities as a service.
Modern vector databases that are brimming with advancements empower you to architect resilient enterprise solutions. Here, we list 15 key features to consider when choosing a vector DB. Every feature may not be important for your use case, but it might be a good place to start. Keep in mind that this area is changing fast, so there might be more features emerging in the future:
- Indexing: As mentioned earlier, indexing refers to the process of organizing highly dimensional vectors in a way that allows for efficient similarity searches and retrievals. A vector DB offers built-in indexing features designed to arrange highly dimensional vectors for swift and effective similarity-based searches and retrievals. Previously, we discussed indexing algorithms such as FAISS and HNSW. Many vector DBs incorporate such features natively. For instance, Azure AI Search integrates the HNSW indexing service directly.
- Search and retrieval: Instead of relying on exact matches, as traditional databases do, vector DBs provide vector search capabilities as a service, such as approximate nearest neighbors (ANNs), to quickly find vectors that are roughly the closest to a given input. To quantify the closeness or similarity between vectors, they utilize similarity measures such as cosine similarity or Euclidean distance, enabling efficient and nuanced similarity-based searches in large datasets.
- Create, read, update, and delete: A vector DB manages highly dimensional vectors and offers create, read, update, and delete (CRUD) operations tailored to vectorized data. When vectors are created, they’re indexed for efficient retrieval. Reading often means performing similarity searches to retrieve vectors closest to a given query vector, typically using methods such as ANNs. Vectors can be updated, necessitating potential re-indexing, and they can also be deleted, with the database adjusting its internal structures accordingly to maintain efficiency and consistency.
- Security: This meets GDPR, SOC2 Type II, and HIPAA rules to easily manage access to the console and use SSO. Data is encrypted when stored and in transit, which also provides more granular identity and access management features.
- Serverless: A high-quality vector database is designed to gracefully autoscale with low management overhead as data volumes soar into millions or billions of entries, distributing seamlessly across several nodes. Optimal vector databases grant users the flexibility to adjust the system in response to shifts in data insertion, query frequencies, and underlying hardware configurations.
- Hybrid search: Hybrid search combines traditional keyword-based search methods with other search mechanisms, such as semantic or contextual search, to retrieve results from both the exact term matches and by understanding the underlying intent or context of the query, ensuring a more comprehensive and relevant set of results.
- Semantic re-ranking: This is a secondary ranking step to improve the relevance of search results. It re-ranks the search results that were initially scored by state-of-the-art ranking algorithms such as BM25 and RRF based on language understanding. For instance, Azure AI search employs secondary ranking that uses multi-lingual, deep learning models derived from Microsoft Bing to elevate the results that are most relevant in terms of meaning.
- Auto vectorization/embedding: Auto-embedding in a vector database refers to the automatic process of converting data items into vector representations for efficient similarity searches and retrieval, with access to multiple embedding models.
- Data replication: This ensuresdata availability, redundancy, and recovery in case of failures, safeguarding business continuity and reducing data loss risks.
- Concurrent user access and data isolation: Vector databases support a large number of users concurrently and ensure robust data isolation to ensure updates remain private unless deliberately shared.
- Auto-chunking: Auto-chunking is the automated process of dividing a larger set of data or content into smaller, manageable pieces or chunks for easier processing or understanding. This process helps preserve the semantic relevance of texts and addresses the token limitations of embedding models. We will learn more about chunking strategies in the upcoming sections in this chapter.
- Extensive interaction tools: Prominent vector databases, such as Pinecone, offer versatile APIs and SDKs across languages, ensuring adaptability in integration and management.
- Easy integration: Vector DBs provide seamless integration with LLM orchestration frameworks and SDKs, such as Langchain and Semantic Kernel, and leading cloud providers, such as Azure, GCP, and AWS.
- User-friendly interface: Thisensures an intuitive platform with simple navigation and direct feature access, streamlining the user experience.
- Flexible pricing models: Provides flexible pricing models as per user needs to keep the costs low for the user.
- Low downtime and high resiliency: Resiliency in a vector database (or any database) refers to its ability to recover quickly from failures, maintain data integrity, and ensure continuous availability even in the face of adverse conditions, such as hardware malfunctions, software bugs, or other unexpected disruptions.
As of early 2024, a few prominent open source vector databases include Chroma, Milvus, Quadrant, and Weaviate, while Pinecone and Azure AI search are among the leading proprietary solutions.