Real-life examples of fine-tuning success
In this section, we’ll explore a real-life example of a fine-tuning approach that OpenAI implemented, which yielded remarkable outcomes.
InstructGPT
OpenAI’s InstructGPT is one of the most successful stories of fine-tuned models that laid the foundation of ChatGPT. ChatGPT is said to be a sibling model to InstructGPT. The methods that are used to fine-tune ChatGPT are similar to InstructGPT. InstructGPT was created by fine-tuning pre-trained GPT-3 models with RHLF. Supervised fine-tuning is the first step in RLHF for generating responses aligned to human preferences.
In the beginning, GPT-3 models weren’t originally designed to adhere to user instructions. Their training focused on predicting the next word based on vast amounts of internet text data. Therefore, these models underwent fine-tuning using instructional datasets along with RLHF to enhance their ability to generate more useful and relevant responses aligned with human values when prompted with user instructions:
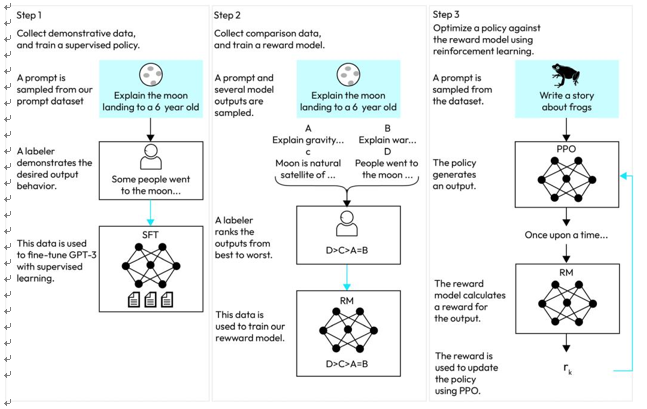
Figure 3.20 – The fine-tuning process with RLHF
This figure depicts a schematic representation showcasing the InstructGPT fine- tuning process: (1) initial supervised fine-tuning, (2) training the reward model, and (3) executing RL through PPO using this established reward model. The utilization of this data to train respective models is indicated by the presence of blue arrows. In step 2, boxes A-D are samples from models that get ranked by labelers.
The following figure provides a comparison of the response quality of fine-tuned models with RLHF, supervised fine-tuned models, and general GPT models. The Y-axis consists of a Likert scale and shows quality ratings of model outputs on a 1–7 scale (Y-axis), for various model sizes (X-axis), on prompts submitted to InstructGPT models via the OpenAI API. The results reveal that InstructGPT outputs receive significantly higher scores by labelers compared to outputs from GPT-3 models with both few-shot prompts and those without, as well as models that underwent supervised learning fine-tuning. The labelers that were hired for this work were independent and were sourced from Scale AI and Upwork:
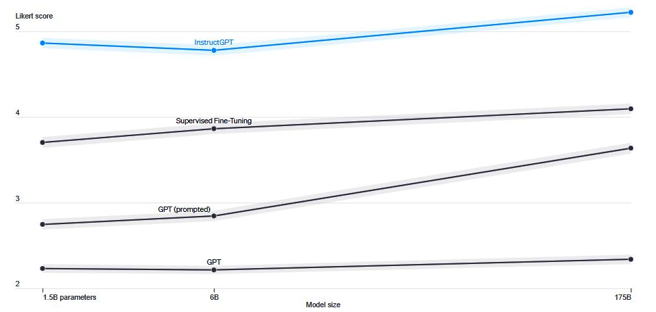
Figure 3.21 – Evaluation of InstructGPT (image credits: Open AI)
InstructGPT can be assessed across dimensions of toxicity, truthfulness, and appropriateness. Higher scores are desirable for TruthfulQA and appropriateness, whereas lower scores are preferred for toxicity and hallucinations. Measurement of hallucinations and appropriateness is conducted based on the distribution of prompts within our API. The outcomes are aggregated across various model sizes:
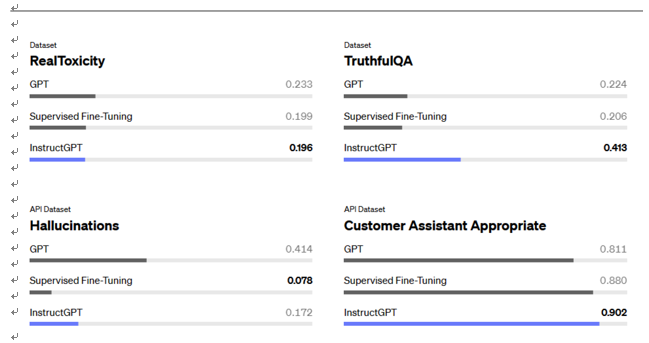
Figure 3.22 – Evaluation of InstructGPT
In this section, we introduced the concept of fine-tuning and discussed a success stories of fine-tuning with RLHF that led to the development of InstructGPT.
Summary
Fine-tuning is a powerful technique for customizing models, but it may not always be necessary. As observed, it can be time-consuming and may have initial upfront costs. It’s advisable to start with easier and faster strategies, such as prompt engineering with few- shot examples, followed by data grounding using RAG. Only if the responses from the LLM remain suboptimal should you consider fine-tuning. We will discuss RAG and prompt engineering in the following chapters.
In this chapter, we delved into critical fine-tuning strategies tailored for specific tasks. Then, we explored an array of evaluation methods and benchmarks to assess your refined model. The RLHF process ensures your models align with human values, making them helpful, honest, and safe. In the upcoming chapter, we’ll tackle RAG methods paired with vector databases – an essential technique to ground your enterprise data and minimize hallucinations in LLM-driven applications.